Teamwork in Big Networks
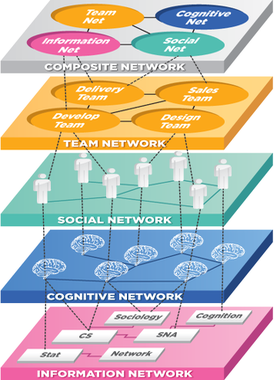
Teams are increasingly indispensable to achievement in any organization. Despite our substantial dependency on teams, fundamental knowledge about the conduct of team-enabled operations is lacking, especially at the social, cognitive and information level in relation to team performance and network dynamics.
The emergence of network science and the advent of big data era create a brand new environment where different users collaborate with each other to collectively perform complex cognitive tasks by providing an easier-than-ever access to the massive knowledge base as well as the broad social connectivity at an unprecedented scale and granularity.
The goal of this project is two-folds:
understand the dynamic associational and causal mechanisms that drive peak team performance
create a suite of new instruments to predict, explore and design high-performing teams
Video demo: [Link]
System entry: [Link]
System tutorial: [Slides]
Source code: [Link]
This project is an on-going multi-disciplinary, multi-institute effort, coordinated by the IDEA Lab@UIUC led by Dr. Hanghang Tong, with contributions from many leading researchers (please refer to the related papers below).
Main Contacts:
Mr. Qinghai Zhou (UIUC, for team optimization and explanation algorithms)
Dr. Liangyue Li (ASU, for team prediction, optimization and explanation algorithms)
Dr. Nan Cao (Tongji University, for system architecture, graph visualization and HCI)
Tutorials & Invited Talks
Liangyue Li, Hanghang Tong. Network Science of Teams: Characterization, Prediction, and Optimization. 24th ACM SIGKDD Conference on Knowledge Discovery and Data Mining (KDD), 2018. [Website]
Liangyue Li, Hanghang Tong. Network Science of Teams: Characterization, Prediction, and Optimization. The 11th ACM International Conference on Web Search and Data Mining (WSDM), 2018.
Hanghang Tong. Towards Optimal Teams in Big Networks. Invited Talk at 2nd International Workshop on Machine Learning Methods for Recommender Systems (MLRec), 2016. [Slides]
Books
Liangyue Li, Hanghang Tong. Computational Approaches to the Network Science of Teams, Cambridge University Press, 2020. (ISBN: 9781108498548)
Journal Papers
Liangyue Li, Hanghang Tong. Network Science of Teams: Current State and Future Tools. IEEE Intelligent Informatics Bulletin (IIB), Vol. 19, No. 2, 2018.
Yong Wang, Conglei Shi, Liangyue Li, Hanghang Tong, Huamin Qu. Visualizing Research Impact Through Citation Data. ACM Transactions on Interactive Intelligent Systems (TIIS), 2017.
Liangyue Li, Hanghang Tong, Nan Cao, Kate Ehrlich, Yu-Ru Lin, Norbou Buchler. Enhancing Team Composition in Professional Networks: Problem Definitions and Fast Solutions. IEEE Transactions on Knowledge and Data Engineering (TKDE), 2016.
Conference Papers
Qinghai Zhou, Liangyue Li, Xintao Wu, Nan Cao, Lei Ying, Hanghang Tong. ATTENT: Active Attributed Network Alignment. 30th The Web Conference (WWW), 2021.
Qinghai Zhou, Liangyue Li, and Hanghang Tong, Towards Real Time Team Optimization. 2019 IEEE International Conference on Big Data (IEEE BigData), 2019.
Qinghai Zhou, Liangyue Li, Nan Cao, Lei Ying, Hanghang Tong. Admiring: Adversarial Multi-Network Mining. 19th IEEE International Conference on Data mining (ICDM), 2019.
Liangyue Li, Hanghang Tong, Huan Liu. Towards Explainable Networked Prediction. The 27th ACM International Conference on Information and Knowledge Management (CIKM), 2018.[PDF]
Liangyue Li, Hanghang Tong, Yong Wang, Conglei Shi, Nan Cao and Norbou Buchler. Is the Whole Greater Than the Sum of Its Parts? 23rd ACM SIGKDD Conference on Knowledge Discovery and Data Mining (KDD), 2017. (Oral Presentation)[PDF] [Slides] [Youtube][Code]
Liangyue Li, How Jing, Hanghang Tong, Jaewon Yang, Qi He and Bee-Chung Chen. NEMO: Next Career Move Prediction with Contextual Embedding. 26th International World Wide Web Conference (WWW), 2017. (Industry Track)[PDF][Slides]
Liangyue Li, Yuan Yao, Jie Tang, Wei Fan, Hanghang Tong. QUINT: On Query-Specific Optimal Networks. 22nd ACM SIGKDD Conference on Knowledge Discovery and Data Mining (KDD), 2016. (Full Presentation)[PDF] [Slides][Code][Youtube]
Liangyue Li, Hanghang Tong, Jie Tang, Wei Fan. iPath: Forecasting the Pathway to Impact. SIAM International Conference on Data Mining (SDM), 2016. [PDF][Slides]
Liangyue Li, Hanghang Tong. The Child is Father of the Man: Foresee the Success at the Early Stage. 21st ACM SIGKDD Conference on Knowledge Discovery and Data Mining (KDD), 2015. (Research Track) [PDF][Slides]
Liangyue Li, Hanghang Tong, Nan Cao, Kate Ehrlich, Yu-Ru Lin, Norbou Buchler. Replacing the Irreplaceable: Fast Algorithm for Team Member Recommendation. International World Wide Web Conference (WWW), 2015. (Acceptance Rate: 14.1%) [PDF]
Nan Cao, Yu-Ru Lin, Liangyue Li, Hanghang Tong. g-Miner: Interactive Visual Group Mining on Multivariate Graphs.
ACM Conference on Human Factors in Computing Systems (CHI), 2015. [PDF]
Liangyue Li, Hanghang Tong, Yanghua Xiao, Wei Fan. Cheetah: Fast Graph Kernel Tracking on Dynamic Graphs. SIAM International Conference on Data Mining (SDM), 2015. [PDF]
Yuan Yao, Hanghang Tong, Feng Xu, Jian Lu. Predicting long-term impact of CQA posts: a comprehensive viewpoint. ACM SIGKDD International Conference on Knowledge Discovery and Data Mining (KDD), 2014. [PDF]
Yuan Yao, Hanghang Tong, Xifeng Yan, Feng Xu, Jian Lu. Multi-Aspect \(+\) Transitivity \(+\) Bias: An Integral Trust Inference Model. IEEE Trans. Knowl. Data Eng. 26(7): 1706-1719 (2014). [PDF]
Danai Koutra, Hanghang Tong, David Lubensky. BIG-ALIGN: Fast Bipartite Graph Alignment. International Conference on Data Mining (ICDM), 2013. [PDF]
U. Kang, Hanghang Tong, Jimeng Sun. Fast Random Walk Graph Kernel. SIAM International Conference on Data Mining (SDM), 2012. [PDF]
Jingrui He, Hanghang Tong, Qiaozhu Mei, Boleslaw K. Szymanski. GenDeR: A Generic Diversified Ranking Algorithm. Annual Conference on Neural Information Processing Systems (NIPS), 2012. [PDF]
Hanghang Tong, Jingrui He, Zhen Wen, Ravi Konuru, Ching-Yung Lin. Diversified ranking on large graphs: an optimization viewpoint. ACM SIGKDD International Conference on Knowledge Discovery and Data Mining (KDD), 2011. [PDF]
Demos and Workshop Papers
Qinghai Zhou, Liangyue Li, Nan Cao, Norbou Buchler and Hanghang Tong. EXTRA: Explaining Team Recommendation in Networks. 12th ACM Conference on Recommender Systems (RecSys), 2018. (Demo Track)
Liangyue Li, Hanghang Tong, Nan Cao, Kate Ehrlich, Yu-Ru Lin and Norbou Buchler. TEAMOPT: Interactive Team Optimization in Big Networks. 25th ACM International Conference on Information and Knowledge Management (CIKM), 2016. (Demo Track) [PDF][Demo]
Liangyue Li, Hanghang Tong, Nan Cao, Kate Ehrlich, Yu-Ru Lin and Norbou Buchler. Enhancing Team Composition in
Professional Networks: Problem Definitions and Fast Solutions. Poster at Science of Team Science (SciTS) Conference 2016.
Liangyue Li, Hanghang Tong, Nan Cao, Kate Ehrlich, Yu-Ru Lin and Norbou Buchler. Computational Methods to Enhance Team Composition in Networks. Poster at the 2nd International Computational Social Science (IC2S2) Conference, 2016.
|